Examples#
All the examples have a download link at the end. You can load the example’s notebook on Google Colab and run them by adding the line
pip install git+https://github.com/deepinv/deepinv.git#egg=deepinv
to the top of the notebook (e.g., as in here).
Basics#
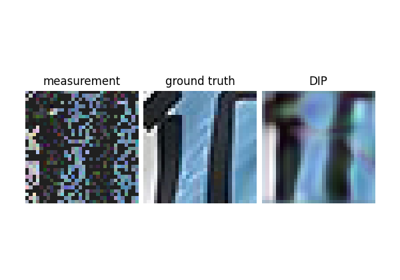
Reconstructing an image using the deep image prior.
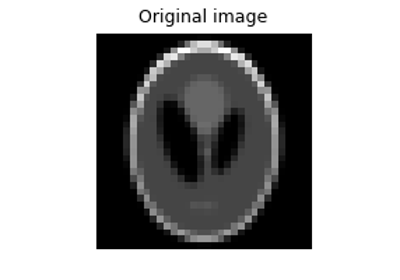
Random phase retrieval and reconstruction methods.
Optimization#
Plug-and-Play#
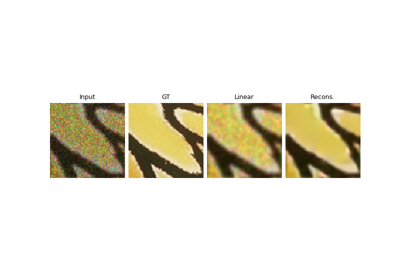
Plug-and-Play algorithm with Mirror Descent for Poisson noise inverse problems.
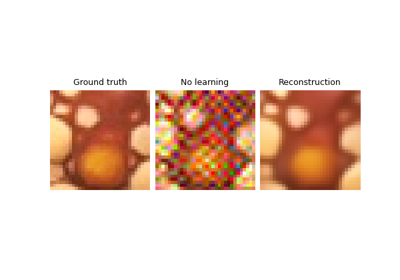
Regularization by Denoising (RED) for Super-Resolution.
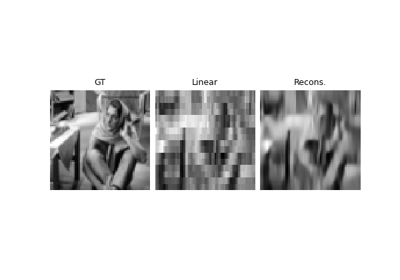
PnP with custom optimization algorithm (Condat-Vu Primal-Dual)
Sampling#
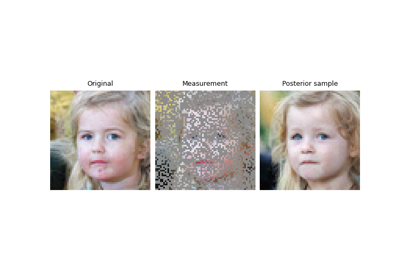
Posterior Sampling for Inverse Problems with Stochastic Differential Equations modeling.
Unfolded#
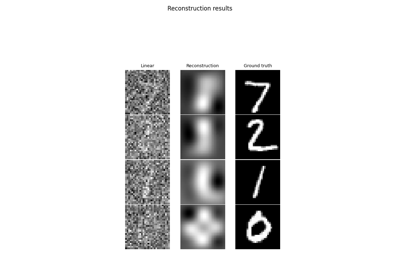
Learned Iterative Soft-Thresholding Algorithm (LISTA) for compressed sensing
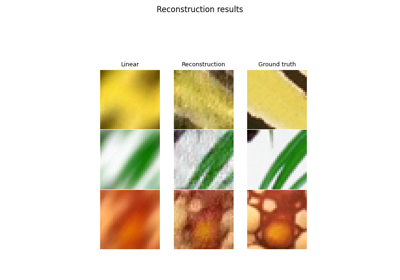
Deep Equilibrium (DEQ) algorithms for image deblurring
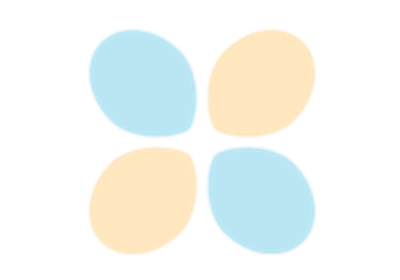
Unfolded Chambolle-Pock for constrained image inpainting
Patch Priors#
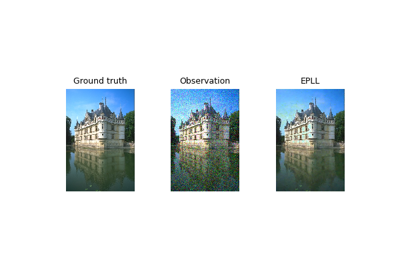
Expected Patch Log Likelihood (EPLL) for Denoising and Inpainting
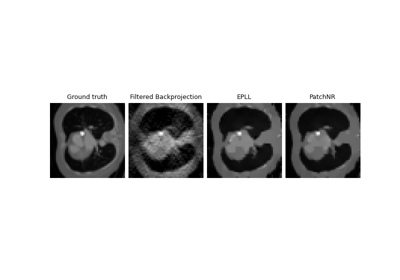
Patch priors for limited-angle computed tomography
Self-Supervised Learning#
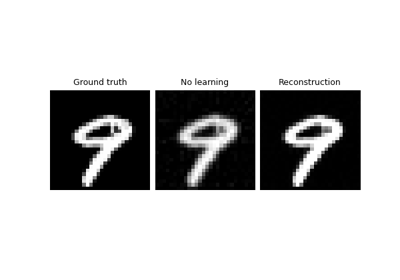
Self-supervised learning with measurement splitting
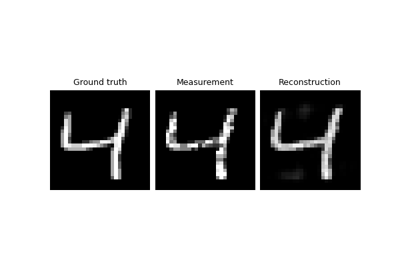
Self-supervised denoising with the Neighbor2Neighbor loss.
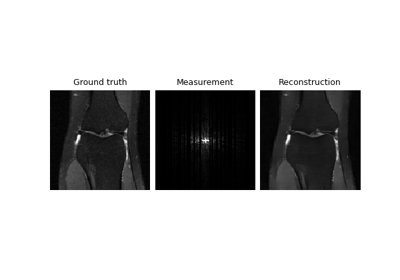
Self-supervised learning with Equivariant Imaging for MRI.
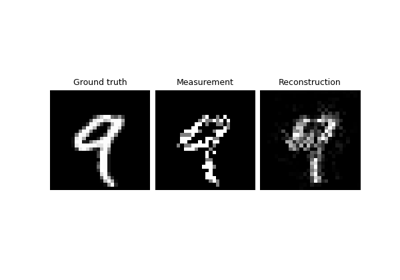
Self-supervised denoising with the Generalized R2R loss.
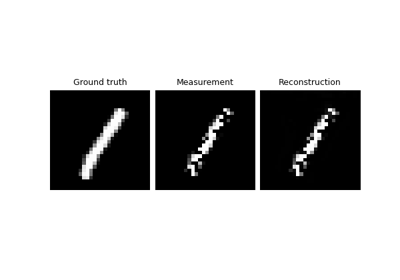
Self-supervised learning from incomplete measurements of multiple operators.
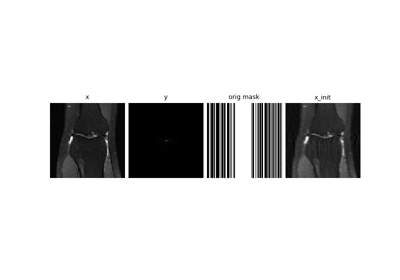
Self-supervised MRI reconstruction with Artifact2Artifact
Adversarial Learning#
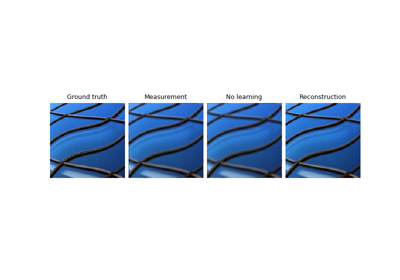
Imaging inverse problems with adversarial networks