Note
Go to the end to download the full example code.
Self-supervised learning with Equivariant Imaging for MRI.#
This example shows you how to train a reconstruction network for an MRI inverse problem on a fully self-supervised way, i.e., using measurement data only.
The equivariant imaging loss is presented in “Equivariant Imaging: Learning Beyond the Range Space”.
from pathlib import Path
import torch
from torch.utils.data import DataLoader
from torchvision import transforms
import deepinv as dinv
from deepinv.datasets import SimpleFastMRISliceDataset
from deepinv.utils import get_data_home, load_degradation
from deepinv.models.utils import get_weights_url
from deepinv.models import MoDL
Setup paths for data loading and results.#
BASE_DIR = Path(".")
DATA_DIR = BASE_DIR / "measurements"
CKPT_DIR = BASE_DIR / "ckpts"
# Set the global random seed from pytorch to ensure reproducibility of the example.
torch.manual_seed(0)
device = dinv.utils.get_freer_gpu() if torch.cuda.is_available() else "cpu"
Load base image datasets and degradation operators.#
In this example, we use a mini demo subset of the single-coil FastMRI dataset as the base image dataset, consisting of 2 knee images of size 320x320.
See also
- Datasets
deepinv.datasets.FastMRISliceDataset
deepinv.datasets.SimpleFastMRISliceDataset
We provide convenient datasets to easily load both raw and reconstructed FastMRI images. You can download more data on the FastMRI site.
Important
By using this dataset, you confirm that you have agreed to and signed the FastMRI data use agreement.
Note
We reduce to the size to 128x128 for faster training in the demo.
operation = "MRI"
img_size = 128
transform = transforms.Compose([transforms.Resize(img_size)])
train_dataset = SimpleFastMRISliceDataset(
get_data_home(), transform=transform, train_percent=0.5, train=True, download=True
)
test_dataset = SimpleFastMRISliceDataset(
get_data_home(), transform=transform, train_percent=0.5, train=False
)
0%| | 0/820529 [00:00<?, ?it/s]
100%|██████████| 801k/801k [00:00<00:00, 68.4MB/s]
Generate a dataset of knee images and load it.#
mask = load_degradation("mri_mask_128x128.npy")
# defined physics
physics = dinv.physics.MRI(mask=mask, device=device)
# Use parallel dataloader if using a GPU to speed up training,
# otherwise, as all computes are on CPU, use synchronous data loading.
num_workers = 4 if torch.cuda.is_available() else 0
n_images_max = (
900 if torch.cuda.is_available() else 5
) # number of images used for training
my_dataset_name = "demo_equivariant_imaging"
measurement_dir = DATA_DIR / "fastmri" / operation
deepinv_datasets_path = dinv.datasets.generate_dataset(
train_dataset=train_dataset,
test_dataset=test_dataset,
physics=physics,
device=device,
save_dir=measurement_dir,
train_datapoints=n_images_max,
num_workers=num_workers,
dataset_filename=str(my_dataset_name),
)
train_dataset = dinv.datasets.HDF5Dataset(path=deepinv_datasets_path, train=True)
test_dataset = dinv.datasets.HDF5Dataset(path=deepinv_datasets_path, train=False)
mri_mask_128x128.npy degradation downloaded in datasets
Dataset has been saved at measurements/fastmri/MRI/demo_equivariant_imaging0.h5
Set up the reconstruction network#
As a (static) reconstruction network, we use an unrolled network
(half-quadratic splitting) with a trainable denoising prior based on the
DnCNN architecture which was proposed in MoDL.
See deepinv.models.MoDL
for details.
Set up the training parameters#
We choose a self-supervised training scheme with two losses: the measurement consistency loss (MC) and the equivariant imaging loss (EI). The EI loss requires a group of transformations to be defined. The forward model should not be equivariant to these transformations. Here we use the group of 4 rotations of 90 degrees, as the accelerated MRI acquisition is not equivariant to rotations (while it is equivariant to translations).
See docs for full list of available transforms.
Note
We use a pretrained model to reduce training time. You can get the same results by training from scratch for 150 epochs using a larger knee dataset of ~1000 images.
epochs = 1 # choose training epochs
learning_rate = 5e-4
batch_size = 16 if torch.cuda.is_available() else 1
# choose self-supervised training losses
# generates 4 random rotations per image in the batch
losses = [dinv.loss.MCLoss(), dinv.loss.EILoss(dinv.transform.Rotate(n_trans=4))]
# choose optimizer and scheduler
optimizer = torch.optim.Adam(model.parameters(), lr=learning_rate, weight_decay=1e-8)
scheduler = torch.optim.lr_scheduler.StepLR(optimizer, step_size=int(epochs * 0.8) + 1)
# start with a pretrained model to reduce training time
file_name = "new_demo_ei_ckp_150_v3.pth"
url = get_weights_url(model_name="demo", file_name=file_name)
ckpt = torch.hub.load_state_dict_from_url(
url,
map_location=lambda storage, loc: storage,
file_name=file_name,
)
# load a checkpoint to reduce training time
model.load_state_dict(ckpt["state_dict"])
optimizer.load_state_dict(ckpt["optimizer"])
Downloading: "https://huggingface.co/deepinv/demo/resolve/main/new_demo_ei_ckp_150_v3.pth?download=true" to /home/runner/.cache/torch/hub/checkpoints/new_demo_ei_ckp_150_v3.pth
0%| | 0.00/2.17M [00:00<?, ?B/s]
100%|██████████| 2.17M/2.17M [00:00<00:00, 113MB/s]
Train the network#
verbose = True # print training information
wandb_vis = False # plot curves and images in Weight&Bias
train_dataloader = DataLoader(
train_dataset, batch_size=batch_size, num_workers=num_workers, shuffle=True
)
test_dataloader = DataLoader(
test_dataset, batch_size=batch_size, num_workers=num_workers, shuffle=False
)
# Initialize the trainer
trainer = dinv.Trainer(
model,
physics=physics,
epochs=epochs,
scheduler=scheduler,
losses=losses,
optimizer=optimizer,
train_dataloader=train_dataloader,
plot_images=True,
device=device,
save_path=str(CKPT_DIR / operation),
verbose=verbose,
wandb_vis=wandb_vis,
show_progress_bar=False, # disable progress bar for better vis in sphinx gallery.
ckp_interval=10,
)
model = trainer.train()
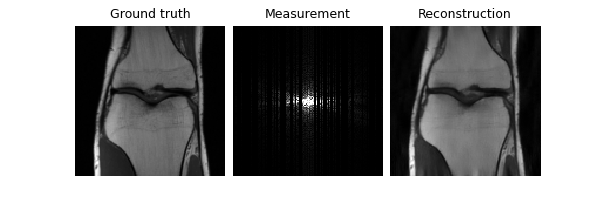
The model has 187019 trainable parameters
Train epoch 0: MCLoss=0.0, EILoss=0.0, TotalLoss=0.0, PSNR=40.568
Test the network#
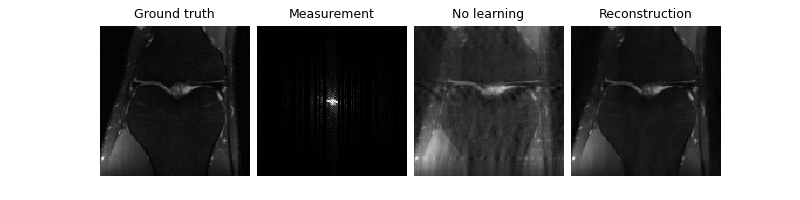
Eval epoch 0: PSNR=37.439, PSNR no learning=32.749
Test results:
PSNR no learning: 32.749 +- 0.000
PSNR: 37.439 +- 0.000
{'PSNR no learning': np.float64(32.74856948852539), 'PSNR no learning_std': 0, 'PSNR': np.float64(37.43928527832031), 'PSNR_std': 0}
Total running time of the script: (0 minutes 11.630 seconds)