Trainer#
- class deepinv.Trainer(model, physics, optimizer, train_dataloader, ...)[source]#
Bases:
object
Trainer class for training a reconstruction network.
See also
See the User Guide for more details and for how to adapt the trainer to your needs.
Training can be done by calling the
deepinv.Trainer.train()
method, whereas testing can be done by calling thedeepinv.Trainer.test()
method.Training details are saved every
ckp_interval
epochs in the following formatsave_path/yyyy-mm-dd_hh-mm-ss/ckp_{epoch}.pth.tar
where
.pth.tar
file contains a dictionary with the keys:epoch
current epoch,state_dict
the state dictionary of the model,loss
the loss history,optimizer
the state dictionary of the optimizer, andeval_metrics
the evaluation metrics history.Assuming that
x
is the ground-truth reference andy
is the measurement andparams
is a dict of physics parameters, the dataloaders should return one of the following options:(x, y)
or(x, y, params)
, which requiresonline_measurements=False
(default) otherwisey
will be ignored and new measurements will be generated online.(x)
or(x, params)
, which requiresonline_measurements=True
for generating measurements in an online manner (optionally with parameters) asy=physics(x)
ory=physics(x, **params)
. Otherwise, first generate a dataset of(x,y)
withdeepinv.datasets.generate_dataset
and then use option 1 above.If you have a dataset of measurements only
(y)
or(y, params)
you should modify it such that it returns(torch.nan, y)
or(torch.nan, y, params)
. Setonline_measurements=False
.
Note
The losses and evaluation metrics can be chosen from our training losses or our metrics
Custom losses can be used, as long as it takes as input
(x, x_net, y, physics, model)
and returns a tensor of lengthbatch_size
(i.e.reduction=None
in the underlying metric, as we perform averaging to deal with uneven batch sizes), wherex
is the ground truth,x_net
is the network reconstruction \(\inversef{y}{A}\),y
is the measurement vector,physics
is the forward operator andmodel
is the reconstruction network. Note that not all inputs need to be used by the loss, e.g., self-supervised losses will not make use ofx
.Custom metrics can also be used in the exact same way as custom losses.
Note
The training code can synchronize with Weights & Biases for logging and visualization by setting
wandb_vis=True
. The user can also customize the wandb setup by providing a dictionary with the setup for wandb.Parameters are described below, grouped into Basics, Optimization, Evaluation, Physics Generators, Model Saving, Comparing with Pseudoinverse Baseline, Plotting, Verbose and Weights & Biases.
- Basics:
- Parameters:
model (deepinv.models.Reconstructor, torch.nn.Module) – Reconstruction network, which can be any reconstruction network. or any other custom reconstruction network.
physics (deepinv.physics.Physics, list[deepinv.physics.Physics]) – Forward operator(s).
train_dataloader (torch.utils.data.DataLoader, list[torch.utils.data.DataLoader]) – Train data loader(s), see options 1 to 3 above for how we expect data to be provided.
online_measurements (bool) – Generate new measurements
y
in an online manner at each iteration by callingy=physics(x)
. IfFalse
(default), the measurements are loaded from the training dataset.device (str, torch.device) – Device on which to run the training (e.g., ‘cuda’ or ‘cpu’). Default is ‘cuda’ if available, otherwise ‘cpu’.
- Optimization:
- Parameters:
optimizer (None, torch.optim.Optimizer) – Torch optimizer for training the network. Default is
None
.epochs (int) – Number of training epochs. Default is 100. The trainer will perform gradient steps equal to the
min(epochs*n_batches, max_batch_steps)
.max_batch_steps (int) – Number of gradient steps per iteration. Default is
1e10
. The trainer will perform batch steps equal to themin(epochs*n_batches, max_batch_steps)
.scheduler (None, torch.optim.lr_scheduler.LRScheduler) – Torch scheduler for changing the learning rate across iterations. Default is
None
.early_stop (bool) – If
True
, the training stops when the evaluation metric is not improving. Default isFalse
. The user can modify the strategy for saving the best model by overriding thedeepinv.Trainer.stop_criterion()
method.losses (deepinv.loss.Loss, list[deepinv.loss.Loss]) – Loss or list of losses used for training the model. Optionally wrap losses using a loss scheduler for more advanced training. See the libraries’ training losses. Where relevant, the underlying metric should have
reduction=None
as we perform the averaging usingdeepinv.utils.AverageMeter
to deal with uneven batch sizes. Default issupervised loss
.grad_clip (float) – Gradient clipping value for the optimizer. If None, no gradient clipping is performed. Default is None.
optimizer_step_multi_dataset (bool) – If
True
, the optimizer step is performed once on all datasets. IfFalse
, the optimizer step is performed on each dataset separately.
- Evaluation:
- Parameters:
eval_dataloader (None, torch.utils.data.DataLoader, list[torch.utils.data.DataLoader]) – Evaluation data loader(s), see options 1 to 3 above for how we expect data to be provided.
metrics (Metric, list[Metric]) – Metric or list of metrics used for evaluating the model. They should have
reduction=None
as we perform the averaging usingdeepinv.utils.AverageMeter
to deal with uneven batch sizes. See the libraries’ evaluation metrics. Default isPSNR
.eval_interval (int) – Number of epochs (or train iters, if
log_train_batch=True
) between each evaluation of the model on the evaluation set. Default is1
.log_train_batch (bool) – if
True
, log train batch and eval-set metrics and losses for each train batch during training. This is useful for visualising train progress inside an epoch, not just over epochs. IfFalse
(default), log average over dataset per epoch (standard training).
Tip
If a validation dataloader
eval_dataloader
is provided, the trainer will also save the best model according to the first metric in the list, using the following format:save_path/yyyy-mm-dd_hh-mm-ss/ckp_best.pth.tar
. The user can modify the strategy for saving the best model by overriding thedeepinv.Trainer.save_best_model()
method. The best model can be also loaded using thedeepinv.Trainer.load_best_model()
method.
- Physics Generators:
- Parameters:
physics_generator (None, deepinv.physics.generator.PhysicsGenerator) – Optional physics generator for generating the physics operators. If not
None
, the physics operators are randomly sampled at each iteration using the generator. Should be used in conjunction withonline_measurements=True
, no effect whenonline_measurements=False
. Also seeloop_random_online_physics
. Default isNone
.loop_random_online_physics (bool) – if
True
, resets the physics generator and noise model back to its initial state at the beginning of each epoch, so that the same measurements are generated each epoch. Requiresshuffle=False
in dataloaders. IfFalse
, generates new physics every epoch. Used in conjunction withonline_measurements=True
andphysics_generator
or noise model inphysics
, no effect whenonline_measurements=False
. Default isFalse
.
Warning
If the physics changes at each iteration for online measurements (e.g. if
physics_generator
is used to generate random physics operators or noise model is used), the generated measurements will randomly vary each epoch. If this is not desired (i.e. you want the same online measurements each epoch), setloop_random_online_physics=True
. This resets the physics generator and noise model’s random generators every epoch.Caveat: this requires
shuffle=False
in your dataloaders.An alternative, safer solution is to generate and save params offline using
deepinv.datasets.generate_dataset()
. The params dict will then be automatically updated every time data is loaded.
- Model Saving:
- Parameters:
- Comparison with Pseudoinverse Baseline:
- Parameters:
compare_no_learning (bool) – If
True
, the no learning method is compared to the network reconstruction. Default isFalse
.no_learning_method (str) – Reconstruction method used for the no learning comparison. Options are
'A_dagger'
,'A_adjoint'
,'prox_l2'
, or'y'
. Default is'A_dagger'
. The user can also provide a custom method by overriding theno_learning_inference
method. Default is'A_dagger'
.
- Plotting:
- Parameters:
plot_images (bool) – Plots reconstructions every
ckp_interval
epochs. Default isFalse
.plot_measurements (bool) – Plot the measurements y, default=`True`.
plot_convergence_metrics (bool) – Plot convergence metrics for model, default=`False`.
rescale_mode (str) – Rescale mode for plotting images. Default is
'clip'
.
- Verbose:
- Parameters:
verbose (bool) – Output training progress information in the console. Default is
True
.verbose_individual_losses (bool) – If
True
, the value of individual losses are printed during training. Otherwise, only the total loss is printed. Default isTrue
.show_progress_bar (bool) – Show a progress bar during training. Default is
True
.check_grad (bool) – Compute and print the gradient norm at each iteration. Default is
False
.display_losses_eval (bool) – If
True
, the losses are displayed during evaluation. Default isFalse
.
- Weights & Biases:
- Parameters:
wandb_vis (bool) – Logs data onto Weights & Biases, see https://wandb.ai/ for more details. Default is
False
.wandb_setup (dict) – Dictionary with the setup for wandb, see https://docs.wandb.ai/quickstart for more details. Default is
{}
.plot_interval (int) – Frequency of plotting images to wandb during train evaluation (at the end of each epoch). If
1
, plots at each epoch. Default is1
.freq_plot (int) – deprecated. Use
plot_interval
- compute_loss(physics, x, y, train=True, epoch=None, step=False)[source]#
Compute the loss and perform the backward pass.
It evaluates the reconstruction network, computes the losses, and performs the backward pass.
- Parameters:
physics (deepinv.physics.Physics) – Current physics operator.
x (torch.Tensor) – Ground truth.
y (torch.Tensor) – Measurement.
train (bool) – If
True
, the model is trained, otherwise it is evaluated.epoch (int) – current epoch.
step (bool) – Whether to perform an optimization step when computing the loss.
- Returns:
(tuple) The network reconstruction x_net (for plotting and computing metrics) and the logs (for printing the training progress).
- compute_metrics(x, x_net, y, physics, logs, train=True, epoch=None)[source]#
Compute the metrics.
It computes the metrics over the batch.
- Parameters:
x (torch.Tensor) – Ground truth.
x_net (torch.Tensor) – Network reconstruction.
y (torch.Tensor) – Measurement.
physics (deepinv.physics.Physics) – Current physics operator.
logs (dict) – Dictionary containing the logs for printing the training progress.
train (bool) – If
True
, the model is trained, otherwise it is evaluated.epoch (int) – current epoch.
- Returns:
The logs with the metrics.
- get_samples(iterators, g)[source]#
Get the samples.
This function returns a dictionary containing necessary data for the model inference. It needs to contain the measurement, the ground truth, and the current physics operator, but can also contain additional data.
- get_samples_offline(iterators, g)[source]#
Get the samples for the offline measurements.
In this setting, samples have been generated offline and are loaded from the dataloader. This function returns a tuple containing necessary data for the model inference. It needs to contain the measurement, the ground truth, and the current physics operator, but can also contain additional data (you can override this function to add custom data).
If the dataloader returns 3-tuples, this is assumed to be
(x, y, params)
whereparams
is a dict of physics generator params. These params are then used to update the physics.
- get_samples_online(iterators, g)[source]#
Get the samples for the online measurements.
In this setting, a new sample is generated at each iteration by calling the physics operator. This function returns a dictionary containing necessary data for the model inference. It needs to contain the measurement, the ground truth, and the current physics operator, but can also contain additional data.
- load_best_model()[source]#
Load the best model.
It loads the model from the checkpoint saved during training.
- Returns:
The model.
- log_metrics_wandb(logs, step, train=True)[source]#
Log the metrics to wandb.
It logs the metrics to wandb.
- model_inference(y, physics, x=None, train=True, **kwargs)[source]#
Perform the model inference.
It returns the network reconstruction given the samples.
- Parameters:
y (torch.Tensor) – Measurement.
physics (deepinv.physics.Physics) – Current physics operator.
x (torch.Tensor) – Optional ground truth, used for computing convergence metrics.
- Returns:
The network reconstruction.
- no_learning_inference(y, physics)[source]#
Perform the no learning inference.
By default it returns the (linear) pseudo-inverse reconstruction given the measurement.
- Parameters:
y (torch.Tensor) – Measurement.
physics (deepinv.physics.Physics) – Current physics operator.
- Returns:
Reconstructed image.
- plot(epoch, physics, x, y, x_net, train=True)[source]#
Plot and optinally save the reconstructions.
- Parameters:
epoch (int) – Current epoch.
physics (deepinv.physics.Physics) – Current physics operator.
x (torch.Tensor) – Ground truth.
y (torch.Tensor) – Measurement.
x_net (torch.Tensor) – Network reconstruction.
train (bool) – If
True
, the model is trained, otherwise it is evaluated.
- save_best_model(epoch, train_ite, **kwargs)[source]#
Save the best model using validation metrics.
By default, uses validation based on first metric. Override this method to provide custom criterion.
- save_model(filename, epoch, state={})[source]#
Save the model.
It saves the model every
ckp_interval
epochs.
- setup_train(train=True, **kwargs)[source]#
Set up the training process.
It initializes the wandb logging, the different metrics, the save path, the physics and dataloaders, and the pretrained checkpoint if given.
- Parameters:
train (bool) – whether model is being trained.
- step(epoch, progress_bar, train_ite=None, train=True, last_batch=False)[source]#
Train/Eval a batch.
It performs the forward pass, the backward pass, and the evaluation at each iteration.
- Parameters:
epoch (int) – Current epoch.
progress_bar – tqdm progress bar.
train_ite (int) – train iteration, only needed for logging if
Trainer.log_train_batch=True
train (bool) – If
True
, the model is trained, otherwise it is evaluated.last_batch (bool) – If
True
, the last batch of the epoch is being processed.
- Returns:
The current physics operator, the ground truth, the measurement, and the network reconstruction.
- stop_criterion(epoch, train_ite, **kwargs)[source]#
Stop criterion for early stopping.
By default, stops optimization when first eval metric doesn’t improve in the last 3 evaluations.
Override this method to early stop on a custom condition.
- Parameters:
epoch (int) – Current epoch.
train_ite (int) – Current training batch iteration, equal to (current epoch \(\times\) number of batches) + current batch within epoch
metric_history (dict) – Dictionary containing the metrics history, with the metric name as key.
metrics (list) – List of metrics used for evaluation.
- test(test_dataloader, save_path=None, compare_no_learning=True, log_raw_metrics=False)[source]#
Test the model, compute metrics and plot images.
- Parameters:
test_dataloader (torch.utils.data.DataLoader, list[torch.utils.data.DataLoader]) – Test data loader(s), see options 1 to 3 above for how we expect data to be provided.
save_path (str) – Directory in which to save the plotted images.
compare_no_learning (bool) – If
True
, the linear reconstruction is compared to the network reconstruction.log_raw_metrics (bool) – if
True
, also return non-aggregated metrics as a list.
- Returns:
dict of metrics results with means and stds.
- Return type:
Examples using Trainer
:#
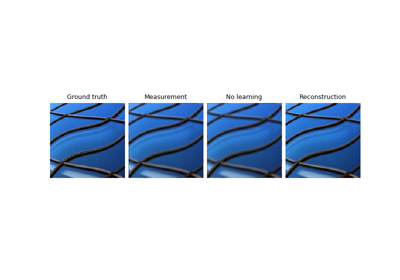
Imaging inverse problems with adversarial networks
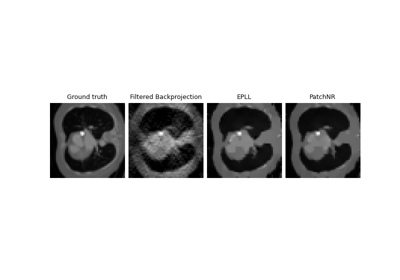
Patch priors for limited-angle computed tomography
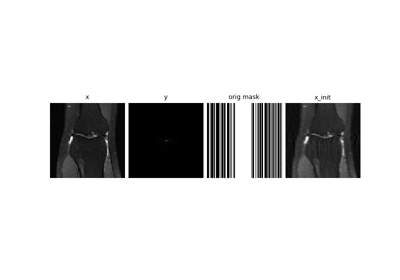
Self-supervised MRI reconstruction with Artifact2Artifact
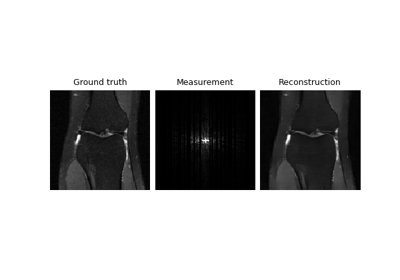
Self-supervised learning with Equivariant Imaging for MRI.
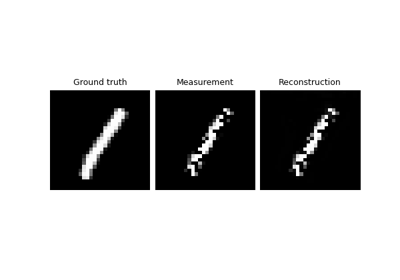
Self-supervised learning from incomplete measurements of multiple operators.
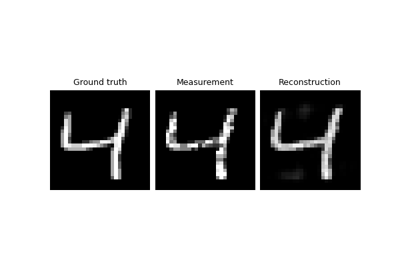
Self-supervised denoising with the Neighbor2Neighbor loss.
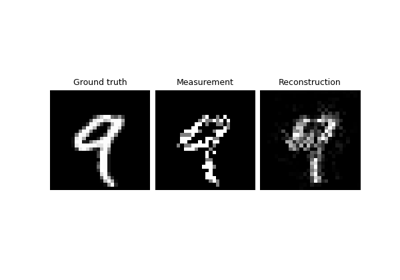
Self-supervised denoising with the Generalized R2R loss.
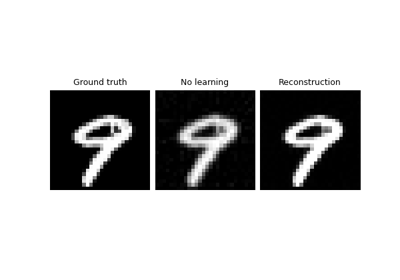
Self-supervised learning with measurement splitting
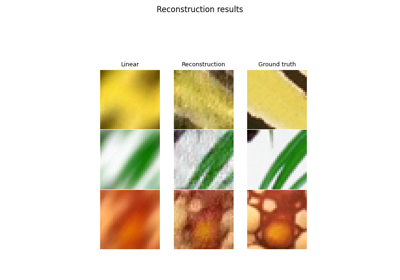
Deep Equilibrium (DEQ) algorithms for image deblurring
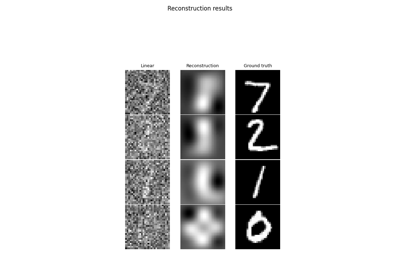
Learned Iterative Soft-Thresholding Algorithm (LISTA) for compressed sensing
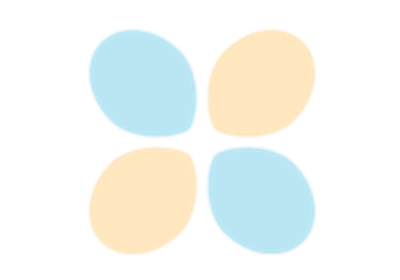
Unfolded Chambolle-Pock for constrained image inpainting