BaseSDESolver#
- class deepinv.sampling.BaseSDESolver(timesteps, rng=None)[source]#
Bases:
Module
Base class for solving Stochastic Differential Equations (SDEs) from
deepinv.sampling.BaseSDE
of the form:\[d x_{t} = f(x_t, t) dt + g(t) d w_{t}\]where \(f\) is the drift term, \(g\) is the diffusion coefficient, and \(w_t\) is a standard Brownian process.
Currently only supported for fixed time steps for numerical integration.
- Parameters:
timesteps (torch.Tensor, numpy.ndarray, list) – time steps at which the SDE will be discretized.e.
rng (torch.Generator) – a random number generator for reproducibility, optional.
- randn_like(input, seed=None)[source]#
Equivalent to
torch.randn_like()
but supports a pseudorandom number generator argument.- Parameters:
input (torch.Tensor) – The input tensor whose size will be used.
seed (int) – The seed for the random number generator, if
rng
is provided.
- Returns:
A tensor of the same size as input filled with random numbers from a normal distribution.
- Return type:
This method uses the
rng
attribute of the class, which is a pseudo-random number generator for reproducibility. If a seed is provided, it will be used to set the state ofrng
before generating the random numbers.Note
The
rng
attribute must be initialized for this method to work properly.
- rng_manual_seed(seed=None)[source]#
Sets the seed for the random number generator.
- Parameters:
seed (int) – the seed to set for the random number generator. If not provided, the current state of the random number generator is used. Note: it will be ignored if the random number generator is not initialized.
- sample(sde, x_init, seed=None, *args, timesteps=None, get_trajectory=False, **kwargs)[source]#
Solve the Stochastic Differential Equation (SDE) with given time steps.
This function iteratively applies the SDE solver step for each time interval defined by the provided timesteps.
- Parameters:
sde (deepinv.sampling.BaseSDE) – the SDE to solve.
x_init (torch.Tensor) – The initial state of the system.
seed (int) – The seed for the random number generator, if
rng
is provided.timesteps (torch.Tensor, numpy.ndarray, list) – A sequence of time points at which to solve the SDE. If None, default timesteps will be used.
get_trajectory (bool) – whether to return the full trajectory of the SDE or only the last sample, optional. Default to False.
*args – Variable length argument list to be passed to the step function.
**kwargs – Arbitrary keyword arguments to be passed to the step function.
- Returns:
SDEOutput
- Return type:
- step(sde, t0, t1, x0, *args, **kwargs)[source]#
Perform a single step with step size from time
t0
to timet1
, with current statex0
.- Parameters:
sde (deepinv.sampling.BaseSDE) – the SDE to solve.
t0 (float or torch.Tensor) – Time at the start of the step, of size (,).
t1 (float or torch.Tensor) – Time at the end of the step, of size (,).
x0 (torch.Tensor) – Current state of the system, of size (batch_size, d).
- Returns:
Updated state of the system after the step.
- Return type:
Examples using BaseSDESolver
:#
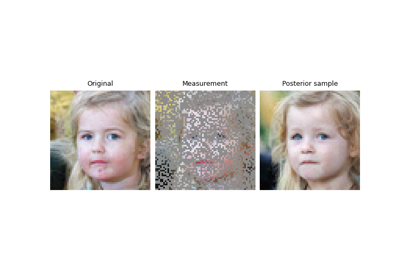
Posterior Sampling for Inverse Problems with Stochastic Differential Equations modeling.