BlurFFT#
- class deepinv.physics.BlurFFT(img_size, filter=None, device='cpu', **kwargs)[source]#
Bases:
DecomposablePhysics
FFT-based blur operator.
It performs the operation
\[y = w*x\]where \(*\) denotes convolution and \(w\) is a filter.
Blur operator based on
torch.fft
operations, which assumes a circular padding of the input, and allows for the singular value decomposition viadeepinv.Physics.DecomposablePhysics
and has fast pseudo-inverse and prox operators.- Parameters:
img_size (tuple) – Input image size in the form (C, H, W).
filter (torch.Tensor) – torch.Tensor of size (1, c, h, w) containing the blur filter with h<=H, w<=W and c=1 or c=C e.g.,
deepinv.physics.blur.gaussian_blur()
.device (str) – cpu or cuda
- Examples:
BlurFFT operator with a basic averaging filter applied to a 16x16 black image with a single white pixel in the center:
>>> from deepinv.physics import BlurFFT >>> x = torch.zeros((1, 1, 16, 16)) # Define black image of size 16x16 >>> x[:, :, 8, 8] = 1 # Define one white pixel in the middle >>> filter = torch.ones((1, 1, 2, 2)) / 4 # Basic 2x2 filter >>> physics = BlurFFT(filter=filter, img_size=(1, 16, 16)) >>> y = physics(x) >>> y[y<1e-5] = 0. >>> y[:, :, 7:10, 7:10] # Display the center of the blurred image tensor([[[[0.2500, 0.2500, 0.0000], [0.2500, 0.2500, 0.0000], [0.0000, 0.0000, 0.0000]]]])
- update_parameters(filter=None, **kwargs)[source]#
Updates the current filter.
- Parameters:
filter (torch.Tensor) – New filter to be applied to the input image.
Examples using BlurFFT
:#
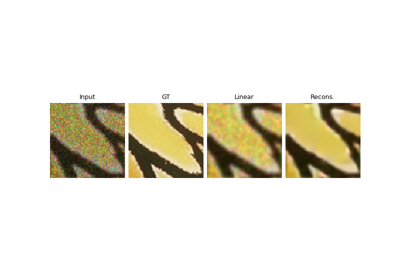
Plug-and-Play algorithm with Mirror Descent for Poisson noise inverse problems.
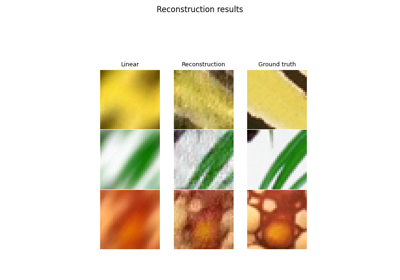
Deep Equilibrium (DEQ) algorithms for image deblurring