GaussianNoise#
- class deepinv.physics.GaussianNoise(sigma=0.1, rng: Generator | None = None)[source]#
Bases:
NoiseModel
Gaussian noise \(y=z+\epsilon\) where \(\epsilon\sim \mathcal{N}(0,I\sigma^2)\).
- Examples:
Adding gaussian noise to a physics operator by setting the
noise_model
attribute of the physics operator:>>> from deepinv.physics import Denoising, GaussianNoise >>> import torch >>> physics = Denoising() >>> physics.noise_model = GaussianNoise() >>> x = torch.rand(1, 1, 2, 2) >>> y = physics(x)
- Parameters:
sigma (float) – Standard deviation of the noise.
rng (torch.Generator (Optional)) – a pseudorandom random number generator for the parameter generation.
- forward(x, sigma=None, seed=None, **kwargs)[source]#
Adds the noise to measurements x
- Parameters:
x (torch.Tensor) – measurements
sigma (float, torch.Tensor) – standard deviation of the noise. If not None, it will overwrite the current noise level.
seed (int) – the seed for the random number generator, if rng is provided.
- Returns:
noisy measurements
- update_parameters(sigma=None, **kwargs)[source]#
Updates the standard deviation of the noise.
- Parameters:
sigma (float, torch.Tensor) – standard deviation of the noise.
Examples using GaussianNoise
:#
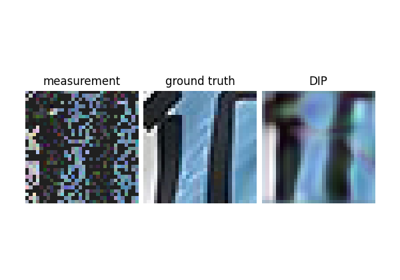
Reconstructing an image using the deep image prior.
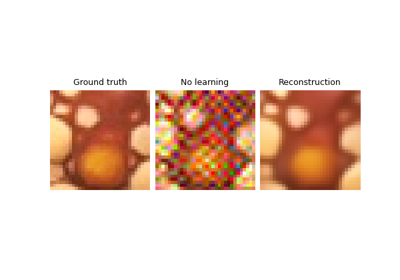
Regularization by Denoising (RED) for Super-Resolution.
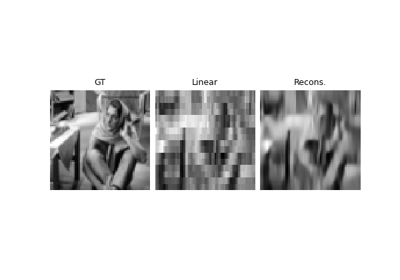
PnP with custom optimization algorithm (Condat-Vu Primal-Dual)
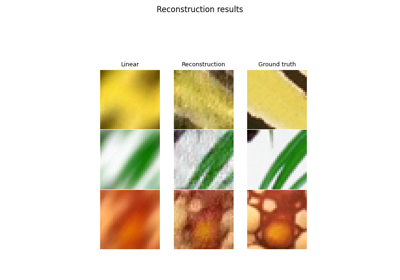
Deep Equilibrium (DEQ) algorithms for image deblurring
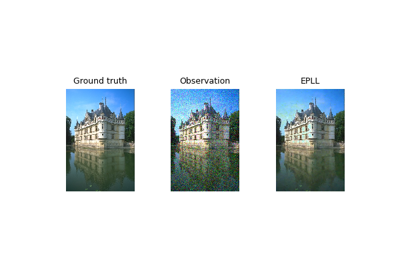
Expected Patch Log Likelihood (EPLL) for Denoising and Inpainting
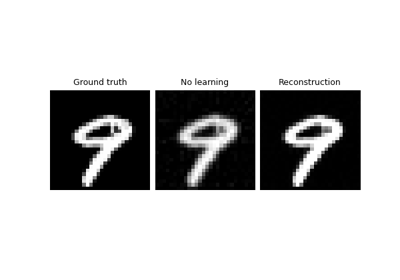
Self-supervised learning with measurement splitting
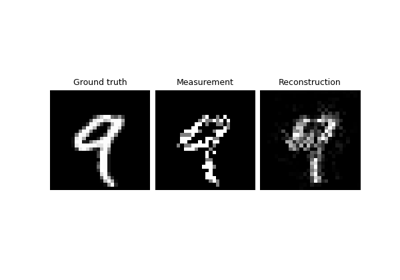
Self-supervised denoising with the Generalized R2R loss.