save_videos#
- deepinv.utils.save_videos(vid_list, titles=None, time_dim=2, rescale_mode='min_max', figsize=None, save_fn=None, **plot_kwargs)[source]#
Saves an animation of a list of image sequences.
Plots videos as sequence of side-by-side frames, and saves animation (e.g. GIF) or displays as interactive HTML in notebook. This is useful for e.g. time-varying inverse problems. Individual frames are plotted with
deepinv.utils.plot()
vid_list can either be a video or a list of them. A video is defined as images of shape(B,C,H,W)
augmented with a time dimension specified bytime_dim
, e.g. of shape(B,C,T,H,W)
andtime_dim=2
. All videos must be same time-length.Per frame of the videos, this function calls
deepinv.utils.plot()
, see its params to see how the frames are plotted.
- Examples:
Display list of image sequences live in a notebook:
>>> from deepinv.utils import save_videos >>> x = torch.rand((1, 3, 5, 8, 8)) # B,C,T,H,W image sequence >>> y = torch.rand((1, 3, 5, 16, 16)) >>> save_videos([x, y], save_fn="vid.gif") # Save video as GIF
- Parameters:
vid_list (Union[torch.Tensor, list[torch.Tensor]]) – video or list of videos as defined above
titles (Union[str, list[str]]) – titles of images in frame, defaults to
None
time_dim (int) – time dimension of the videos. All videos should have same length in this dimension, or length 1. After indexing this dimension, the resulting images should be of shape
(B,C,H,W)
. Defaults to 2rescale_mode (str) – rescaling mode for
deepinv.utils.plot()
, defaults to “min_max”figsize (tuple[int], None) – size of the figure. If
None
, calculated from size of img list.save_fn (str) – if not
None
, save the animation to this filename. File extension must be provided, noteanim_writer
might have to be specified. Defaults toNone
**plot_kwargs – kwargs to pass to
deepinv.utils.plot()
Examples using save_videos
:#
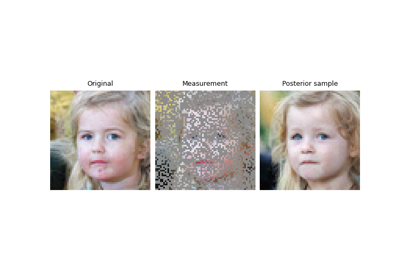
Building your diffusion posterior sampling method using SDEs