VarianceExplodingDiffusion#
- class deepinv.sampling.VarianceExplodingDiffusion(denoiser=None, sigma_min=0.005, sigma_max=100, alpha=1.0, solver=None, dtype=torch.float64, device=torch.device('cpu'), *args, **kwargs)[source]#
Bases:
DiffusionSDE
Variance-Exploding Stochastic Differential Equation (VE-SDE)
The forward-time SDE is defined as follows:
\[d x_t = g(t) d w_t \quad \mbox{where } g(t) = \sigma_{\mathrm{min}} \left( \frac{\sigma_{\mathrm{max}}}{\sigma_{\mathrm{min}}} \right)^t\]The reverse-time SDE is defined as follows:
\[d x_t = -\frac{1 + \alpha}{2} \sigma_{\mathrm{min}}^2 \left( \frac{\sigma_{\mathrm{max}}}{\sigma_{\mathrm{min}}} \right)^{2t} \nabla \log p_t(x_t) dt + \sqrt{\alpha} \sigma_{\mathrm{min}} \left( \frac{\sigma_{\mathrm{max}}}{\sigma_{\mathrm{min}}} \right)^t d w_t\]where \(\alpha \in [0,1]\) is a constant weighting the diffusion term.
This class is the reverse-time SDE of the VE-SDE, serving as the generation process.
- Parameters:
denoiser (deepinv.models.Denoiser) – a denoiser used to provide an approximation of the score at time \(t\) \(\nabla \log p_t(x_t)\).
sigma_min (float) – the minimum noise level.
sigma_max (float) – the maximum noise level.
alpha (float) – the weighting factor of the diffusion term.
solver (deepinv.sampling.BaseSDESolver) – the solver for solving the SDE.
dtype (torch.dtype) – data type of the computation, except for the
denoiser
which will usetorch.float32
. We recommend usingtorch.float64
for better stability and less numerical error when solving the SDE in discrete time, since most computation cost is from evaluating thedenoiser
, which will be always computed intorch.float32
.device (torch.device) – device on which the computation is performed.
- sample_init(shape, rng)[source]#
Sample from the initial distribution of the reverse-time diffusion SDE, which is a Gaussian with zero mean and covariance matrix \(\sigma_{max}^2 \operatorname{Id}\).
- Parameters:
shape (tuple) – The shape of the sample to generate
rng (torch.Generator) – Random number generator for reproducibility
- Returns:
A sample from the prior distribution
- Return type:
Examples using VarianceExplodingDiffusion
:#
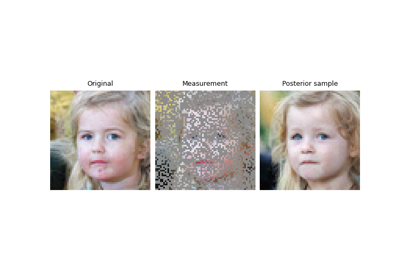
Building your diffusion posterior sampling method using SDEs