L2#
- class deepinv.optim.L2(sigma=1.0)[source]#
Bases:
DataFidelity
Implementation of the data-fidelity as the normalized \(\ell_2\) norm
\[f(x) = \frac{1}{2\sigma^2}\|\forw{x}-y\|^2\]It can be used to define a log-likelihood function associated with additive Gaussian noise by setting an appropriate noise level \(\sigma\).
- Parameters:
sigma (float) – Standard deviation of the noise to be used as a normalisation factor.
>>> import torch >>> import deepinv as dinv >>> # define a loss function >>> fidelity = dinv.optim.data_fidelity.L2() >>> >>> x = torch.ones(1, 1, 3, 3) >>> mask = torch.ones_like(x) >>> mask[0, 0, 1, 1] = 0 >>> physics = dinv.physics.Inpainting(tensor_size=(1, 3, 3), mask=mask) >>> y = physics(x) >>> >>> # Compute the data fidelity f(Ax, y) >>> fidelity(x, y, physics) tensor([0.]) >>> # Compute the gradient of f >>> fidelity.grad(x, y, physics) tensor([[[[0., 0., 0.], [0., 0., 0.], [0., 0., 0.]]]]) >>> # Compute the proximity operator of f >>> fidelity.prox(x, y, physics, gamma=1.0) tensor([[[[1., 1., 1.], [1., 1., 1.], [1., 1., 1.]]]])
- prox(x, y, physics, *args, gamma=1.0, **kwargs)[source]#
Proximal operator of \(\gamma \datafid{Ax}{y} = \frac{\gamma}{2\sigma^2}\|Ax-y\|^2\).
Computes \(\operatorname{prox}_{\gamma \datafidname}\), i.e.
\[\operatorname{prox}_{\gamma \datafidname} = \underset{u}{\text{argmin}} \frac{\gamma}{2\sigma^2}\|Au-y\|_2^2+\frac{1}{2}\|u-x\|_2^2\]- Parameters:
x (torch.Tensor) – Variable \(x\) at which the proximity operator is computed.
y (torch.Tensor) – Data \(y\).
physics (deepinv.physics.Physics) – physics model.
gamma (float) – stepsize of the proximity operator.
- Returns:
(
torch.Tensor
) proximity operator \(\operatorname{prox}_{\gamma \datafidname}(x)\).
Examples using L2
:#
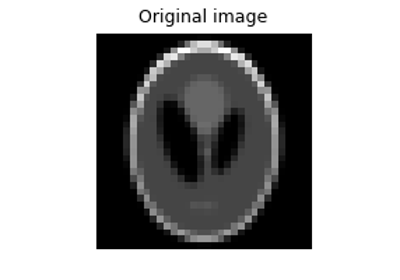
Random phase retrieval and reconstruction methods.
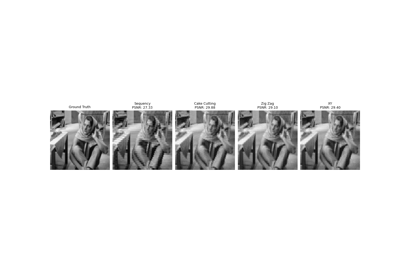
Pattern Ordering in a Compressive Single Pixel Camera
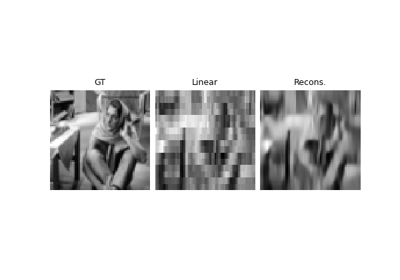
PnP with custom optimization algorithm (Condat-Vu Primal-Dual)
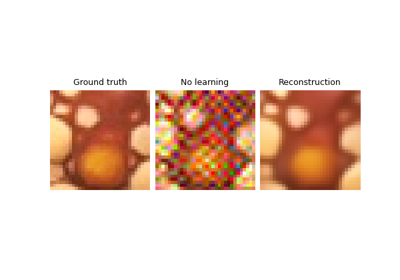
Regularization by Denoising (RED) for Super-Resolution.
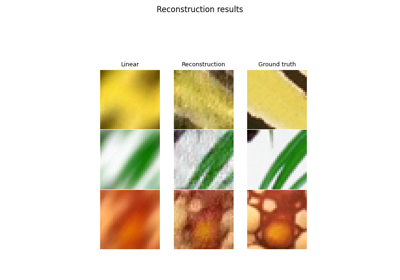
Deep Equilibrium (DEQ) algorithms for image deblurring
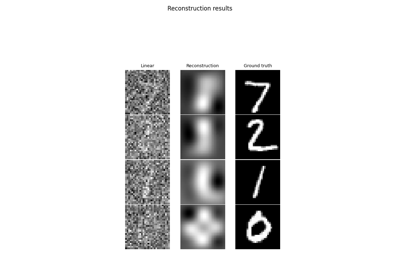
Learned Iterative Soft-Thresholding Algorithm (LISTA) for compressed sensing