Denoiser#
- class deepinv.models.Denoiser(device='cpu')[source]#
Bases:
Module
Base class for denoiser models.
Provides a template for defining denoiser models.
While most denoisers \(\denoisername\) are designed to handle Gaussian noise with variance \(\sigma^2\), this is not mandatory.
Note
A Denoiser can be converted into a
Reconstructor
by using thedeepinv.models.ArtifactRemoval
class.The base class inherits from
torch.nn.Module
.- forward(x, sigma, **kwargs)[source]#
Applies denoiser \(\denoiser{x}{\sigma}\).
- Parameters:
x (torch.Tensor) – noisy input.
sigma (torch.Tensor, float) – noise level.
- Returns:
(
torch.Tensor
) Denoised tensor.
Examples using Denoiser
:#
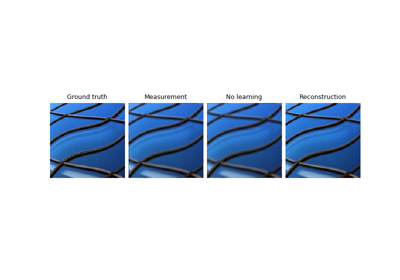
Imaging inverse problems with adversarial networks
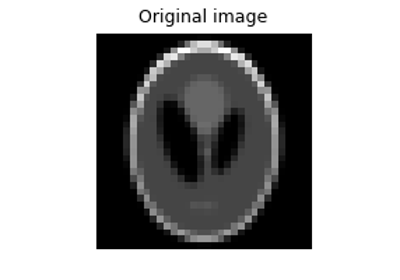
Random phase retrieval and reconstruction methods.
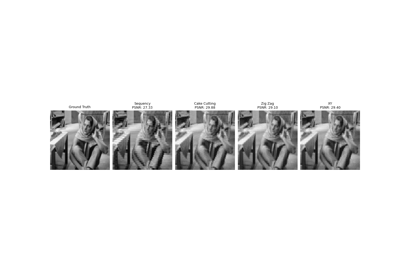
Pattern Ordering in a Compressive Single Pixel Camera
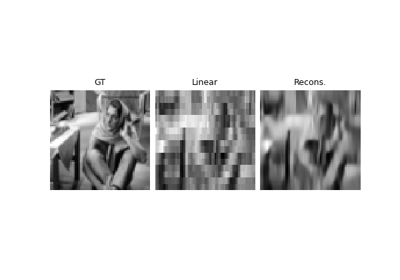
PnP with custom optimization algorithm (Condat-Vu Primal-Dual)
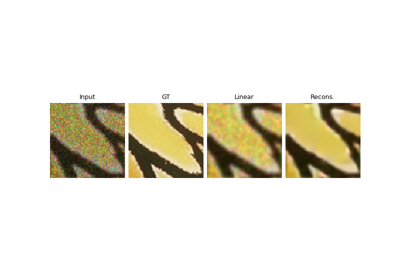
Plug-and-Play algorithm with Mirror Descent for Poisson noise inverse problems.
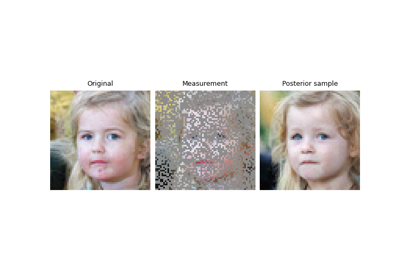
Building your diffusion posterior sampling method using SDEs
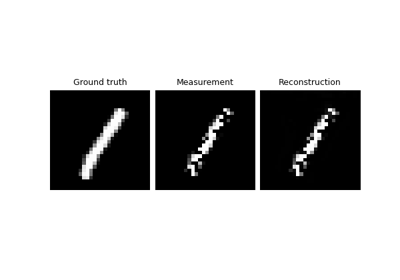
Self-supervised learning from incomplete measurements of multiple operators.
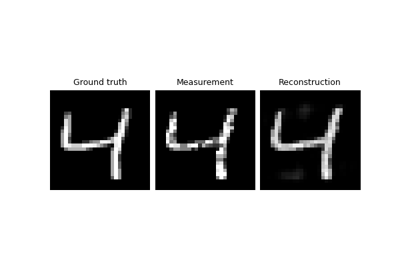
Self-supervised denoising with the Neighbor2Neighbor loss.
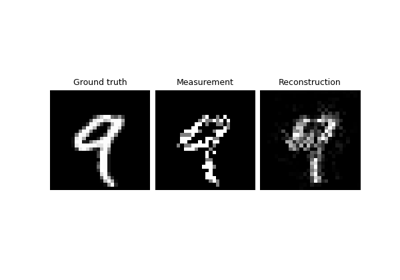
Self-supervised denoising with the Generalized R2R loss.
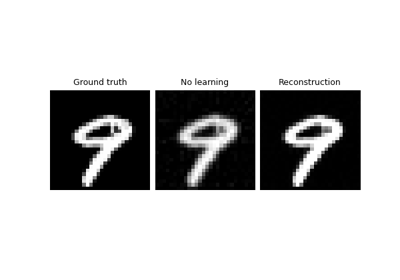
Self-supervised learning with measurement splitting
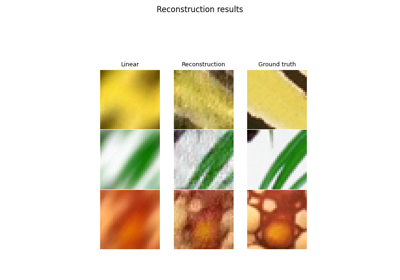
Deep Equilibrium (DEQ) algorithms for image deblurring
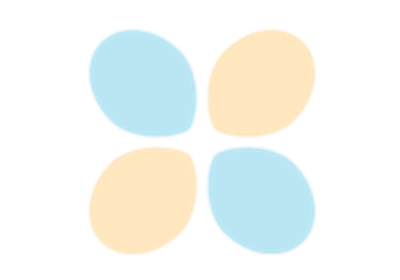
Unfolded Chambolle-Pock for constrained image inpainting