Inpainting#
- class deepinv.physics.Inpainting(img_size, mask=None, pixelwise=True, device='cpu', rng=None, **kwargs)[source]#
Bases:
DecomposablePhysics
Inpainting forward operator, keeps a subset of entries.
The operator is described by the diagonal matrix
\[A = \text{diag}(m) \in \mathbb{R}^{n\times n}\]where \(m\) is a binary mask with \(n\) entries.
This operator is linear and has a trivial SVD decomposition, which allows for fast computation of the pseudo-inverse and proximal operator.
An existing operator can be loaded from a saved
.pth
file viaself.load_state_dict(save_path)
, in a similar fashion totorch.nn.Module
.Masks can also be created on-the-fly using mask generators such as
BernoulliSplittingMaskGenerator
, see example below.- Parameters:
mask (torch.Tensor, float) – If the input is a float, the entries of the mask will be sampled from a bernoulli distribution with probability equal to
mask
. If the input is atorch.Tensor
matchingimg_size
, the mask will be set to this tensor. Ifmask
istorch.Tensor
, it must be shape that is broadcastable to input shape and will be broadcast during forward call. IfNone
, it must be set during forward pass or usingupdate
method.img_size (tuple) – size of the input images without batch dimension e.g. of shape
(C, H, W)
or(C, M)
or(M,)
.device (torch.device) – gpu or cpu.
pixelwise (bool) – Apply the mask in a pixelwise fashion, i.e., zero all channels in a given pixel simultaneously. If existing mask passed (i.e. mask is
torch.Tensor
), this has no effect.rng (torch.Generator) – a pseudorandom random number generator for the mask generation. Default to None.
- Examples:
Inpainting operator using defined mask, removing the second column of a 3x3 image:
>>> from deepinv.physics import Inpainting >>> seed = torch.manual_seed(0) # Random seed for reproducibility >>> x = torch.randn(1, 1, 3, 3) # Define random 3x3 image >>> mask = torch.zeros(1, 3, 3) # Define empty mask >>> mask[:, 2, :] = 1 # Keeping last line only >>> physics = Inpainting(mask=mask, img_size=x.shape[1:]) >>> physics(x) tensor([[[[ 0.0000, -0.0000, -0.0000], [ 0.0000, -0.0000, -0.0000], [ 0.4033, 0.8380, -0.7193]]]])
Inpainting operator using random mask, keeping 70% of the entries of a 3x3 image:
>>> from deepinv.physics import Inpainting >>> seed = torch.manual_seed(0) # Random seed for reproducibility >>> x = torch.randn(1, 1, 3, 3) # Define random 3x3 image >>> physics = Inpainting(mask=0.7, img_size=x.shape[1:]) >>> physics(x) tensor([[[[ 1.5410, -0.0000, -2.1788], [ 0.5684, -0.0000, -1.3986], [ 0.4033, 0.0000, -0.0000]]]])
Generate random masks on-the-fly using mask generators:
>>> from deepinv.physics import Inpainting >>> from deepinv.physics.generator import BernoulliSplittingMaskGenerator >>> x = torch.randn(1, 1, 3, 3) # Define random 3x3 image >>> physics = Inpainting(img_size=x.shape[1:]) >>> gen = BernoulliSplittingMaskGenerator(x.shape[1:], split_ratio=0.7) >>> params = gen.step(batch_size=1, seed = 0) # Generate random mask >>> physics(x, **params) # Set mask on-the-fly tensor([[[[-0.4033, -0.0000, 0.1820], [-0.8567, 1.1006, -1.0712], [ 0.1227, -0.0000, 0.3731]]]]) >>> physics.update(**params) # Alternatively update mask before forward call >>> physics(x) tensor([[[[-0.4033, -0.0000, 0.1820], [-0.8567, 1.1006, -1.0712], [ 0.1227, -0.0000, 0.3731]]]])
- __mul__(other)[source]#
Concatenates two forward operators \(A = A_1\circ A_2\) via the mul operation
If the second operator is an Inpainting or MRI operator, the masks are multiplied element-wise, otherwise the default implementation of LinearPhysics is used (see
deepinv.physics.LinearPhysics.__mul__()
).- Parameters:
other (deepinv.physics.Physics) – Physics operator \(A_2\)
- Returns:
(
deepinv.physics.Physics
) concatenated operator
- noise(x, **kwargs)[source]#
Incorporates noise into the measurements \(\tilde{y} = N(y)\)
- Parameters:
x (torch.Tensor) – clean measurements
- Return torch.Tensor:
noisy measurements
Examples using Inpainting
:#
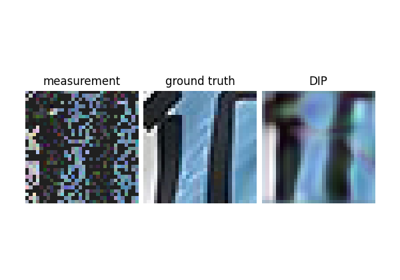
Reconstructing an image using the deep image prior.
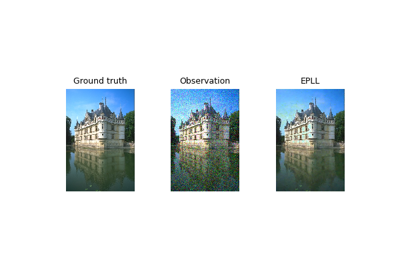
Expected Patch Log Likelihood (EPLL) for Denoising and Inpainting
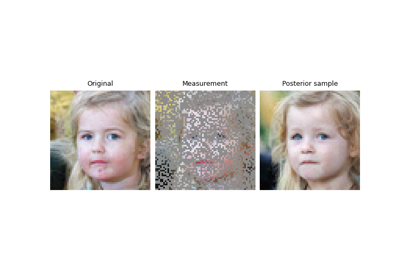
Building your diffusion posterior sampling method using SDEs
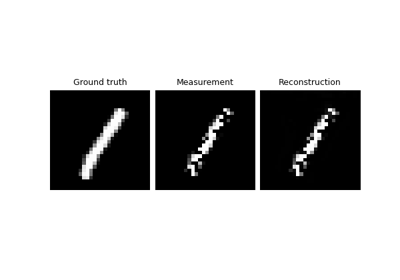
Self-supervised learning from incomplete measurements of multiple operators.
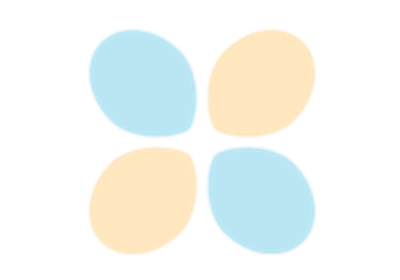
Unfolded Chambolle-Pock for constrained image inpainting